Data Observability
Home > Services > Data, Analytics & AI > Data Governance, Security & Privacy > Data Observability
Data Observability provides comprehensive visibility into the health of your data and data systems. It enables you to detect when data is incorrect, identify what went wrong, and why to help you fix it.
The five pillars of Data Observability—Freshness, Quality, Volume, Schema, and Lineage—offer critical insights into data reliability. Freshness ensures data timeliness, Quality measures accuracy and consistency, Volume tracks completeness, Schema monitors structural integrity, and Lineage provides transparency into data flow and dependencies. Together, these pillars support proactive monitoring, enabling teams to address issues before they impact operations or analytics.
By adopting a robust Data Observability solution, organizations enhance data management, increase team productivity, and improve customer satisfaction through reliable, high-quality data.
Proactive Issue Detection
Identify data issues early, minimizing disruptions to operations.
Enhanced Pipeline Health
Maintain efficient and reliable data flow, reducing downtime.
Improved Productivity
Accelerate root cause analysis, enabling faster issue resolution and better resource utilization.
.Solutions

VSERVE
Revolutionize AI model development with scalable, accurate data enrichment workflows powered by automation for various types of data sets.

IronCloud
Strengthen IT security and compliance across all attack surfaces and industries with centralized management for operational efficiency.

CloudAssist
Centralize and simplify multi-cloud operations with real-time monitoring, planning, budgeting, cost optimization, and automated workflows.
.Client Success
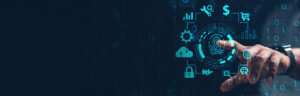
The Integration Challenge Making Sense of Fragmented Cybersecurity Solutions
With an ever-evolving cyber threat landscape, organizations are juggling a growing number of cybersecurity tools and specialized teams to manage them. From basic endpoint detection
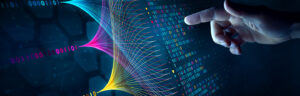
The Evolution of Supervised Learning From Data Labeling to Annotation for RLHF
We humans have experienced forms of supervised learning throughout our lives, starting from hearing “good job” from our parents to receiving “employee of the month” awards at work.
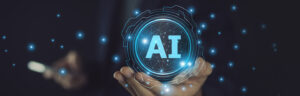
The Evolution of Backup From Tape Libraries to AI Innovation Hubs
In the cloud-native era, backup technology has come a long way. Traditional players like Legato Networker (for those who remember) and Veritas NetBackup